New Imaging Technology Developed by UCLA Research Team May Reduce Need for Skin Biopsies
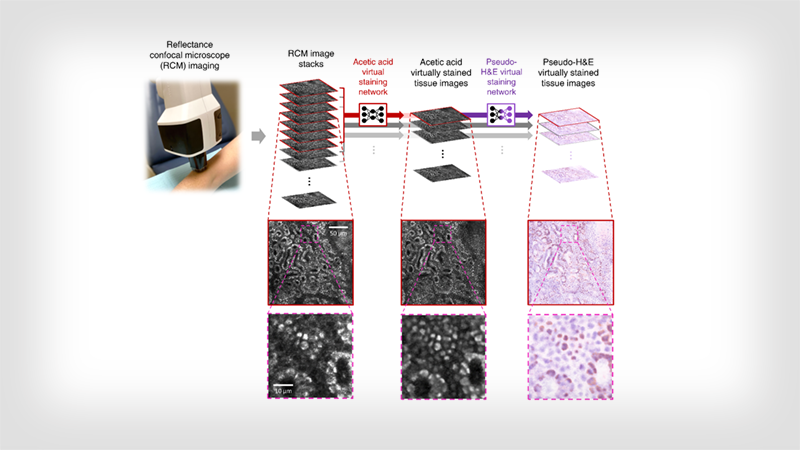
Courtesy of Aydogan Ozcan
UCLA team achieves biopsy-free virtual histology of skin using deep learning and RCM.
This could become routine in clinics, the result of a new “virtual histology” technology being developed by researchers at the UCLA Samueli School of Engineering and the David Geffen School of Medicine at UCLA, according to today’s article in Light: Science & Applications, a journal of the Springer Nature Group. Histology is the study of the microscopic structure of tissues.
The technology, which has been in research and development for more than three years, may provide a new avenue for rapid diagnosis of malignant skin tumors, reducing the number of unnecessary invasive skin biopsies and allowing earlier diagnosis of skin cancer. Previously, this technology has only been applied to microscopy slides that contained unstained tissue, acquired through a biopsy. This report is the first to apply virtual histology to intact, unbiopsied tissue.
The research team, led by Ozcan, Scumpia and Dr. Gennady Rubinstein, a dermatologist at the Dermatology & Laser Centre in Los Angeles, created a deep-learning framework to transform images of intact skin acquired by an emerging noninvasive optical technology, reflectance confocal microscopy (RCM), into a format that is user-friendly for dermatologists and pathologists. Analyzing RCM images requires special training because they are in black and white, and unlike standard histology, they lack nuclear features of cells.
“I was surprised to see how easy it is for this virtual staining technology to transform the images into ones that I typically see of skin biopsies that are processed using traditional chemical fixation and tissue staining under a microscope,” Scumpia said.
The researchers trained a “convolutional neural network” to rapidly transform RCM images of unstained skin into virtually stained 3D images like the H&E (hematoxylin and eosin) images familiar to dermatologists and dermatopathologists. Deep learning, a form of machine learning, constructs artificial neural networks that, like the human brain, can “learn” from large amounts of data.
“This framework can perform virtual histology on a variety of skin conditions, including basal cell carcinoma. It also provides detailed 3D images of several skin layers,” said Ozcan, who also has UCLA faculty appointments in bioengineering and surgery and is an associate director of the California NanoSystems Institute. “In our studies, the virtually stained images showed similar color contrast and spatial features found in traditionally stained microscopic images of biopsied tissue. This approach may allow diagnosticians to see the overall histological features of intact skin without invasive skin biopsies or the time-consuming work of chemical processing and labeling of tissue.”
According to Rubinstein, this is an exciting proof-of-concept study. “The only tool currently used in clinics to help a dermatologist are dermatoscopes, which magnify skin and polarize light. At best, they can help a dermatologist pick up patterns,” said Rubinstein, who also uses reflectance confocal microscopes in clinic.
The authors said several steps remain in translating this technology for clinical use, but their goal is to provide virtual histology technology that can be built into any device — large, small or combined with other optical-imaging systems. Once the neural network is “trained,” with many tissue samples and the use of powerful graphics processing units (GPUs), it will be able to run on a computer or network, enabling rapid transformation from a standard image to a virtual histology image.
Future studies will determine if this digital, biopsy-free approach can interface with whole-body imaging and electronic medical records to usher in a new age of “digital dermatology” and change how dermatology is practiced. Additionally, the research team will determine if this artificial intelligence platform can work with other AI technologies to look for patterns and further aid in clinical diagnosis.
Other authors of this work include electrical and computer engineering assistant adjunct professor Yair Rivenson of UCLA Samueli and Ozcan’s engineering graduate students: Jingxi Li, Jason Garfinkel, Xiaoran Zhang, Di Wu, Yijie Zhang, Kevin de Haan, Hongda Wang, Tairan Liu, and Bijie Bai.
The research is funded by the National Science Foundation, Biophotonics Program (PI: Ozcan). Scumpia, in collaboration with Ozcan, received a VA Merit Award to further study biopsy-free virtual histology in non-melanoma skin cancer in veterans.